upenn adverse impact machine learning testing|Wharton Healthcare Analytics Lab : OEM While ML tools can accurately identify patients at higher risk of short-term adverse outcomes, they are not fortune tellers. About half of patients classified as high risk died within . webRead 60,908 galleries with tag full color on nhentai, a hentai doujinshi and manga reader.
{plog:ftitle_list}
Resultado da Esposarabudarj (novo) Blackpanther1980. 2 70,7K. esposarabudarj (novos) Blackpanther1980. 4 67,5K. esposarabudarj - Hotwife ovascaino777. Esposarabudarj photos & videos. EroMe is the best place to share your erotic pics and porn videos. Every day, thousands of people use EroMe to .
Drug Safety. We have been developing signal detection methods on evaluating vaccine and drug safety using massive Vaccine Adverse Event Reporting System (VAERS) data and FDA .
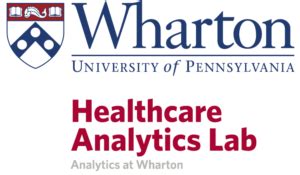
In a new JAMA Oncology study, LDI Senior Fellow Ravi Parikh and colleagues .Growing data availability and advances in machine learning offer a transformative opportunity to deliver effective, dynamic, and customized healthcare. The Wharton Healthcare Analytics Lab (WHAL) develops the . While ML tools can accurately identify patients at higher risk of short-term adverse outcomes, they are not fortune tellers. About half of patients classified as high risk died within . In a new JAMA Oncology study, LDI Senior Fellow Ravi Parikh and colleagues tested whether nudges to clinicians via email and text, prompted by a machine learning algorithm could increase the number of serious illness .
As financial services firms evaluate the potential applications of artificial intelligence (AI), for example: to enhance the customer experience and garner operational efficiencies, Artificial Intelligence/Machine Learning .Adverse Impact Reduction and Job Performance Optimization via Pareto-Optimal Weighting: A Shrinkage Formula and Regularization Technique Using Machine Learning Q. Chelsea Song1, Chen Tang2, Daniel A. Newman3, and Serena Wee4 1 Department of Psychological Sciences, Purdue University 2 Kogod School of Business, American University
For many students, staying awake all night to study is common practice. According to Medical News Today, around 20 percent of students pull all-nighters at least once a month, and about 35 percent stay up past three in the morning once or more weekly.. That being said, staying up all night to study is one of the worst things students can do for their grades. What causes algorithmic bias in health care? How might biases be mitigated? Finding the answers and implementing improvements are the work of the University of Pennsylvania’s Human Algorithm Collaboration lab (HACLab), directed by Master of Health Care Innovation faculty member Ravi B. Parikh, MD, MPP, FACP.. HACLab administers research .
A Machine Learning Approach with Human-AI Collaboration for Automated Classification of Patient Safety Event Reports: Algorithm Development and Validation Study . Adverse events refer to incidents with potential or actual harm to patients in hospitals. These events are typically documented through patient safety event (PSE) reports, which .
1038 LANDERSET AL. and random forest, over traditional regression. Given these results, we suggest the potential of machine learning for employee selection is unlikely to be realized in selectionA committee consisting of faculty from both Penn Engineering and PSOM evaluated 18 applications and judged the proposals based on clinical relevance, AI foundations and potential for impact. Artificial intelligence and machine learning promise to revolutionize nearly every field, sifting through massive amounts of data to find insights that .Michael Kearns1 [email protected] University of Pennsylvania, Philadelphia, PA 19104, USA ————— Portions of this work were conducted while the authors were in the Equity Strategies department of Lehman Brothers in New York City. Appearing in Proceedings of the 23 rd International Conference on Machine Learning, Pittsburgh, PA, 2006.
Employers now have a wide variety of algorithmic decision-making tools available to assist them in making employment decisions, including recruitment, hiring, retention, promotion, transfer, performance monitoring, demotion, dismissal, and referral. Employers increasingly utilize these tools in an attempt to save time and effort, increase objectivity, optimize employee .Keywords: Machine Learning, Big Data, Forecasting. The third author acknowledges financial support from the Fonds de recherche sur la société et la culture (Québec) and the Social Sciences and Humanities Research Council. yCorresponding Author:[email protected]. Department of Economics, UPenn.1 The Adverse Effects of Code Duplication in Machine Learning Models of Code Miltiadis Allamanis Abstract—The field of big code relies on mining large corpora of code to perform some learning task.A significant threat to this approach has been recently identified by Lopes et al. [19] who found a large amount of near-duplicate code on GitHub.machine learning models on them, and release tools to help the community avoid this problem in future research. 1 Introduction Machine learning models of source code have recently re-ceived great attention from the research community. At the .
A study by Liu et al. developed a machine learning model that predicts adverse drug reactions using the chemical, biological, and phenotypic properties of drugs, which demonstrates the potential of machine learning in improving our ability to predict ADRs and drug toxicity [12]. Furthermore, AI and ML can contribute to post-marketing . This study used machine learning to identify predictors of COVID-19 guideline adherence in Denmark to help promote pandemic public health. . Effectiveness of Using Additional HIV Self-Test Kits as an Incentive to Increase HIV Testing Within Assisted Partner Services . University of Pennsylvania 1118 Blockley Hall 423 Guardian Drive . Adversarial testing is a method for systematically evaluating an ML model with the intent of learning how it behaves when provided with malicious or inadvertently harmful input. This guide describes an example adversarial .However, the impact of code duplication has not been noticed by researchers devising machine learning models for source code. In this article, we study the effect of code duplication to machine learning models showing that reported metrics are sometimes inflated by up to .
The purpose of this article is to describe the impact of artificial intelligence (AI), and specifically Machine Learning (ML) and Natural Language Processing (NLP), on personnel selection in terms of potential uses, challenges for practice, and recommendations based on the most recent advances in the science. The United States saw a record 25,487 kidney transplants in 2021, according to the latest annual data report from the Organ Procurement and Transplantation Network and Scientific Registry of Transplant Recipients. Five years after transplantation, successful organ function—called graft survival—of kidneys from deceased donors was 81% among patients .
that is used to train the machine learning model and a test set where the performance of the model is measured. And since duplicated datasets are distributed differently from non-duplicated datasets the machine learning models learn to model a different probability distribution. This is because machine learning makes an important assumption .2021] Machine Learning, Market Manipulation, and Collusion 83 of market manipulation and algorithmic “tacit” collusion.5 Notably, several ethical and legal questions arise when dealing with issues of liability for algorithms’ misbehavior. 6 Our findings suggest that AI’s misconduct can ultimately subvert existing prohibitions ofBy Jonathan Supovitz. Test-based accountability systems — the use of tests to hold individuals or institutions responsible for performance and to reward achievement — have become the cornerstone of U.S. federal education policy, and the past decade has witnessed a widespread adoption of test-based accountability systems in the U.S. Consider just one material .In personnel selection practice, one useful technique for reducing adverse impact and enhancing diversity is the Pareto-optimal weighting approach of De Corte et al. (2007). This approach produces a series of hiring solutions that characterize a diversity–job performance trade-off and can lead to more optimal selection outcomes (sometimes doubling the number of job offers for .

To make our work more reliable, we are comparing the proposed approach with another study [25] toward drug-adverse event extraction using machine learning. The authors in this study reported a .
Wharton Healthcare Analytics Lab
CIS 5190 vs. 5200: Penn CIS offers two different introductory machine learning courses: CIS 4190/5190 (Applied Machine Learning) and CIS 5200 (Machine Learning). While there is overlap, the former (this course!) emphasizes practical application of existing machine learning methods, whereas the latter emphasizes the statistical foundations and . Wharton’s Elizabeth (Zab) Johnson and Michael Platt join Eric Bradlow, vice dean of Analytics at Wharton, to discuss how AI is being used in neuroscience to better understand the human brain.
UNIVERSITY of PENNSYLVANIA CIS 520: Machine Learning Final Exam, 2019 Exam policy: This exam allows one one-page, two-sided cheat sheet; No other materials. Time: 120 minutes. Be sure to write your name and Penn student ID (the 8 bigger digits on your ID card) on the answer form and ll in the associated bubbles in pencil.1.1.2.4 Moderate Data Risk Classification: or Moderate Risk Data Is University of Pennsylvania data where: the data is not generally available to the public; or The loss of confidentiality, integrity, or availability of the data or system could have a mildly adverse impact on the University's mission, safety, finances, or reputation or the loss .

concrete core testing cost

Motherland: Fort Salem é um drama sobrenatural americano criado por Eliot Laurence que estreou na Freeform em 18 de março de 2020. A série é estrelada por Taylor Hickson como Raelle Collar, Jessica Sutton como Tally Craven e Ashley Nicole Williams como Abigail Bellweather, três bruxas recrutadas para o Exército dos EUA. Em maio de 2020, a série foi renovada para uma segunda temporada, que estreou em 22 de junho de 2021. Em agosto de 2021, a série foi reno.
upenn adverse impact machine learning testing|Wharton Healthcare Analytics Lab